Student earns outstanding poster award for machine learning work
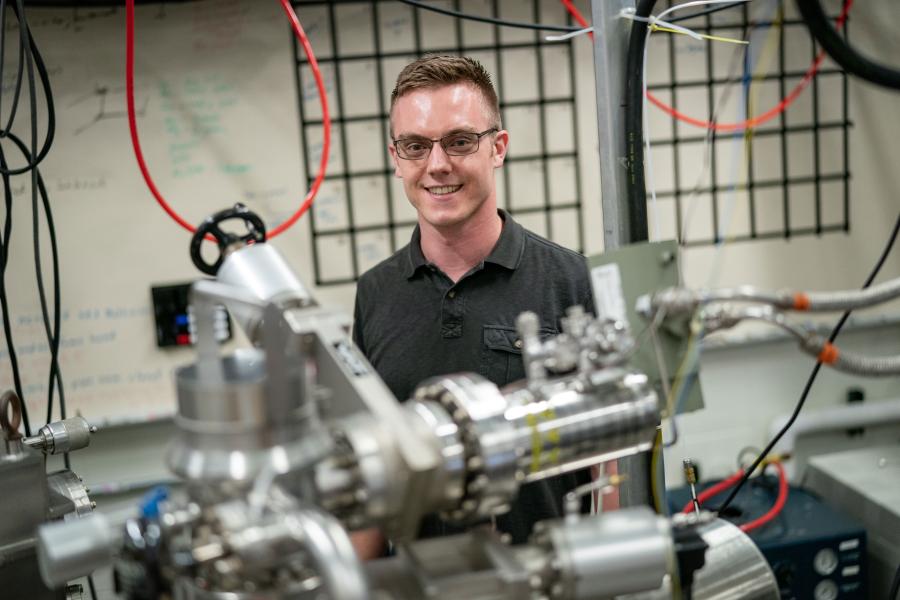
Andrew Messecar, B.S.'18, is a Ph.D. student conducting research in Western's Molecular Beam Epitaxy Lab, housed in the College of Engineering and Applied Sciences.
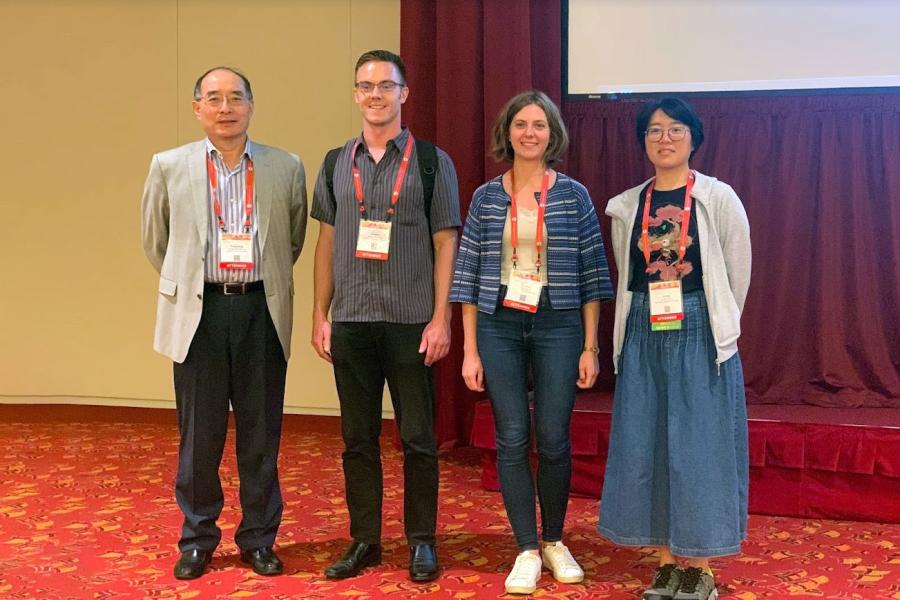
Andrew Messecar, B.S.'18, (second from left) won Outstanding Student Poster Award at the 37th North American Molecular Beam Epitaxy Conference (NAMBE 2023).
The researchers attending the 37th North American Molecular Beam Epitaxy Conference (NAMBE 2023)
KALAMAZOO, Mich.—Western Michigan University doctoral student Andrew Messecar received an Outstanding Student Poster Award at the 37th North American Molecular Beam Epitaxy Conference (NAMBE 2023) where his work focused on using machine learning to study patterns and trends in materials research data.
In his research, Messecar uses machine learning—a process of developing algorithms and statistical models that allow computers to estimate relationships from data. Specifically, he is developing algorithms that recognize patterns in existing research data to determine the best way to use molecular beam epitaxy to grow crystals of electronic materials. These materials and the information being uncovered about how they synthesize will be used to enhance the functionality and performance of next–generation electronic devices. Rather than the traditional trial-and-error approach, which can be both inefficient and expensive, Messecar is looking at ways to better understand how the design of an experiment influences the results.
“Participating in the conference opened up a lot of great connections through conversations with other scientists about how machine learning and data science can be used to analyze and study various physical systems,” says Messecar, whose poster was titled “Machine Learning Analysis and Predictions of Plasma Assisted Molecular Beam Epitaxy III—Nitride Growth.”
The College of Engineering and Applied Sciences houses the Molecular Beam Epitaxy Lab where Messecar conducts research using a technique called plasma–assisted molecular beam epitaxy (PAMBE) to grow thin film crystals of electronic materials. For this project, Messecar studied data from past semiconductor growth experiments using statistical analysis and supervised learning techniques. He first investigated which variables in the growth process have the greatest statistical importance for influencing the end result of a growth trial. With this information, he then used a learning algorithm to predict the experimental outcome of all possible combinations of the two most important synthesis parameters.
“Our goal for using machine learning was to develop a methodology that produces both accurate and physically meaningful predictions. The results that we obtained from this approach indicate that we may have found a methodology that can be optimized and applied to study synthesis–structure relationships of other varieties as well,” he says.
This research is part of Messecar’s dissertation work, which involves using data science and supervised learning techniques, such as machine learning, to study relationships in experimental data describing different physical systems. Some of these projects involve collaborations with research groups at Pennsylvania State University, where he worked as a visiting scholar this past summer.
“I’m ecstatic about the advances in computational and information processing technologies that are currently being developed and the significance that they have for scientific computing, simulation and the study of important phenomena that are often difficult to model. On the experimental side of things, I’m fascinated by the current vein of study that my research group has found themselves in and what results we can develop in the near future to experimentally study synthesis–structure and synthesis–structure–property relationships,” he says.
Messecar’s research is supervised by Dr. Robert Makin, WMU assistant professor of computer science; he also worked on the research with Dr. Steve Durbin, associate dean of engineering at the University of Hawaii. Messecar earned a B.S. in physics from WMU and served as a Peace Corps volunteer from 2018 until 2020 when he returned to Western to pursue his Ph.D.
Learn about developments in Messecar’s research at WMU by following him on LinkedIn.
For more WMU news, arts and events, visit WMU News online.